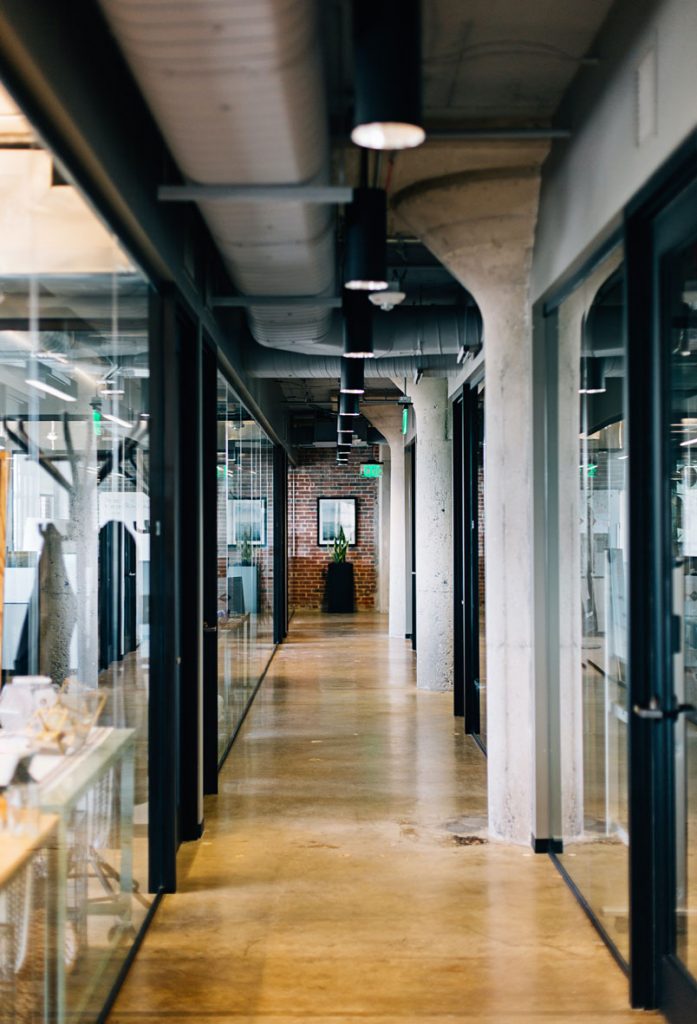
GNoME: A new Deep Learning Tool for Materials Discovery
Materials science is a critical field that underpins countless technological advancements, from the development of new batteries and solar panels to the creation of more efficient electronics. However, the discovery of new materials is often a slow and laborious process, involving extensive experimentation and trial-and-error.
In a recent breakthrough, researchers at Google DeepMind have developed a powerful new deep learning tool called GNoME that has the potential to dramatically accelerate the pace of materials discovery. GNoME utilizes graph neural networks (GNNs) to analyze vast amounts of data on known materials, enabling it to identify promising candidates for further investigation.
Key Takeaways from the GNoME Paper
The paper published in Nature outlines several key takeaways that demonstrate the transformative potential of GNoME:
- Accelerated Materials Discovery: GNoME has been used to discover 2.2 million new crystals, representing a significant leap forward in the field.
- Stable Materials Identification: Among the newly discovered crystals, 380,000 are deemed stable, indicating their potential for practical applications.
- Experimental Validation: External researchers have successfully synthesized 736 of the predicted materials, validating GNoME’s accuracy and reliability.
- State-of-the-Art GNN Model: GNoME leverages a cutting-edge GNN model trained on extensive data from the Materials Project.
- Active Learning Approach: GNoME employs active learning, a technique that continuously improves its performance through iterative learning cycles.
- Cost Reduction and Accelerated Development: GNoME has the potential to reduce the cost of materials discovery and accelerate the development of new technologies.
Implications for the Future of Materials Science
The advent of GNoME marks a significant turning point in materials science. By leveraging the power of deep learning, GNoME holds the promise of revolutionizing the way we discover and develop new materials. With its ability to identify promising candidates, GNoME can guide researchers towards the most fruitful avenues of exploration, streamlining the discovery process and leading to faster advancements in materials science.
Why is it potentially important to me?
The Ski and Outdoor industries have historically, along with the sporting goods manufacturers been very early to spot potential uses for new and novel materials.
For example: A ski base that is potentially harder and more damage resistant, whilst sliding faster, would be a massive win. As would a new waterproof coating that is greener and performs better than existing offerings. This wish list of demands for greater performance and lower environmental cost is one of the reasons why our industry could benefit from the new research.
Conclusion
GNoME represents a remarkable achievement in the field of deep learning and materials science. Its potential to accelerate the discovery of new materials is truly transformative, paving the way for a future filled with innovative materials that will shape the technologies of tomorrow. As GNoME continues to evolve, we can anticipate even greater breakthroughs in materials science, leading to a brighter and more sustainable future. We await the future with hopeful anticipation.